Audio Analysis with Deep Learning: Techniques and Challenges
Welcome to the world of audio analysis, where you can finally learn what your dog has been barking about all these years. But don’t let Fido hog all the spotlight – with deep learning techniques, we can train machines to analyze and understand all kinds of sounds, from the soothing tones of your favorite ASMR video to the ear-splitting screech of a rusty door hinge.
Of course, as with any AI application, there are some challenges to overcome. We can’t just throw any old audio data into our neural networks and hope for the best – we need to carefully curate our datasets to ensure that our models are getting the right training. After all, we don’t want our machine learning algorithms to think that a cat’s meow and a chainsaw revving are the same thing (unless you’re trying to scare off mice, I guess).
But fear not, brave audio enthusiasts! With spectral analysis, convolutional neural networks, and a little bit of ingenuity, we can unlock the full potential of audio analyses with deep learning. Who knows, maybe someday we’ll have machines that can not only classify different sounds, but also identify the exact song that’s been stuck in your head for the past week (and then get it stuck in their own digital heads – sorry, robots).
So buckle up and get ready to dive into the fascinating world of audio analysis with deep learning. Just make sure to keep your headphones on and your volume at a reasonable level – we wouldn’t want to wake up the neighbors (or worse, attract the attention of any malevolent AI overlords).
topic list:
- Unleashing the Potential of Audio Analysis with Deep Learning
- Data Collection and Preprocessing: The First Step to Success
- Spectral Analysis and Convolutional Neural Networks: A Match Made in Audio Heaven
- Beyond Classification: The Challenge of Sound Source Separation
- From Speech Recognition to Music Generation: The Limitless Possibilities of Audio Analysis
- Robustness and Security: The Dark Side of Audio Analysis with Deep Learning
- The Road Ahead: Exploring New Techniques and Applications in Audio Analysis
Unleashing the Potential of Audio Analysis with Deep Learning
Audio analysis has come a long way, from simple spectrograms to advanced neural networks that can identify individual speakers or classify different sound sources. Deep learning has played a major role in this transformation, offering powerful techniques for processing audio data and extracting meaningful features. But along with these exciting new possibilities come a number of challenges that must be overcome in order to make analyses with deep learning truly effective.
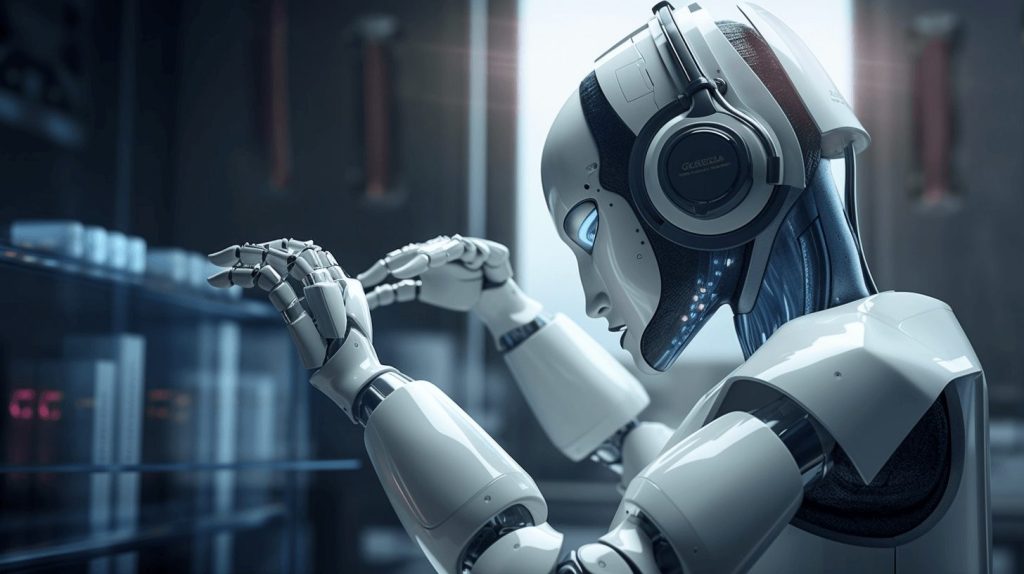
Data Collection and Preprocessing: The First Step to Success
One of the biggest challenges in analysis with deep learning is collecting and preprocessing the right data. Because deep learning models require vast amounts of data to train effectively, researchers must carefully curate their datasets to ensure that they’re relevant, diverse, and representative of the types of sounds the model will be asked to analyze.
Stay up to date on new AI tool releases
Spectral Analysis and Convolutional Neural Networks: A Match Made in Audio Heaven
Spectral analysis is a powerful tool for processing audio signals, and convolutional neural networks (CNNs) are an ideal architecture for extracting meaningful features from those signals. By applying filters to the spectral representation of an audio signal, a CNN can identify patterns and relationships that are difficult for humans to discern, leading to highly accurate sound classification and recognition.
Beyond Classification: The Challenge of Sound Source Separation
While classifying different sounds is an impressive feat, separating individual sound sources within a complex audio signal is an even greater challenge. Sound source separation, which involves separating multiple voices or instruments within a single recording, is an active area of research in audio analysis with deep learning.
From Speech Recognition to Music Generation: The Limitless Possibilities of Audio Analysis
Deep learning models can be used for a wide range of audio analysis tasks, from speech recognition and translation to music generation and remixing. By training models on vast amounts of data and using advanced architectures like recurrent neural networks (RNNs) and generative adversarial networks (GANs), researchers are exploring the frontiers of what’s possible with analyses.
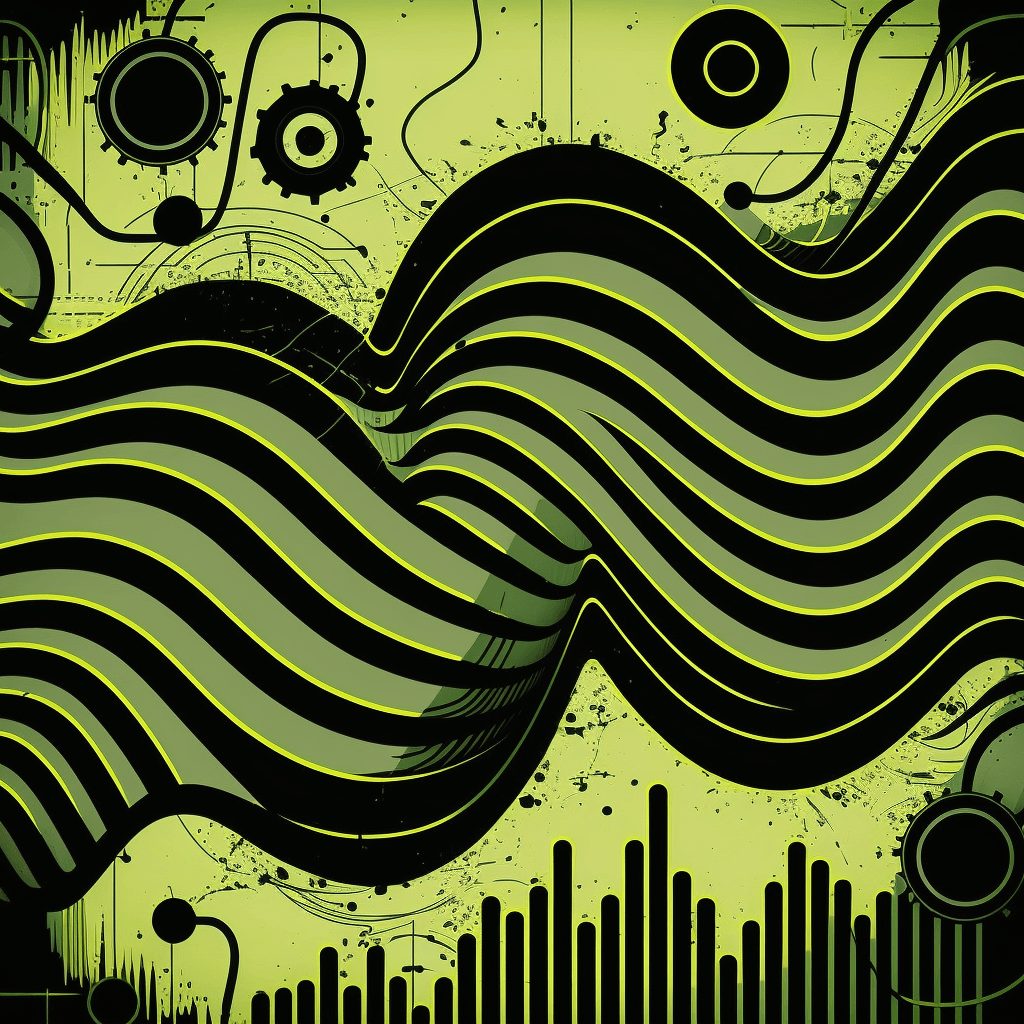
Robustness and Security: The Dark Side of Audio Analysis with Deep Learning
As with any AI application, there are concerns around the robustness and security of deep learning models for audio analysis. Adversarial attacks, which involve manipulating audio signals in subtle ways to fool deep learning models, are a growing area of concern for researchers and practitioners alike.
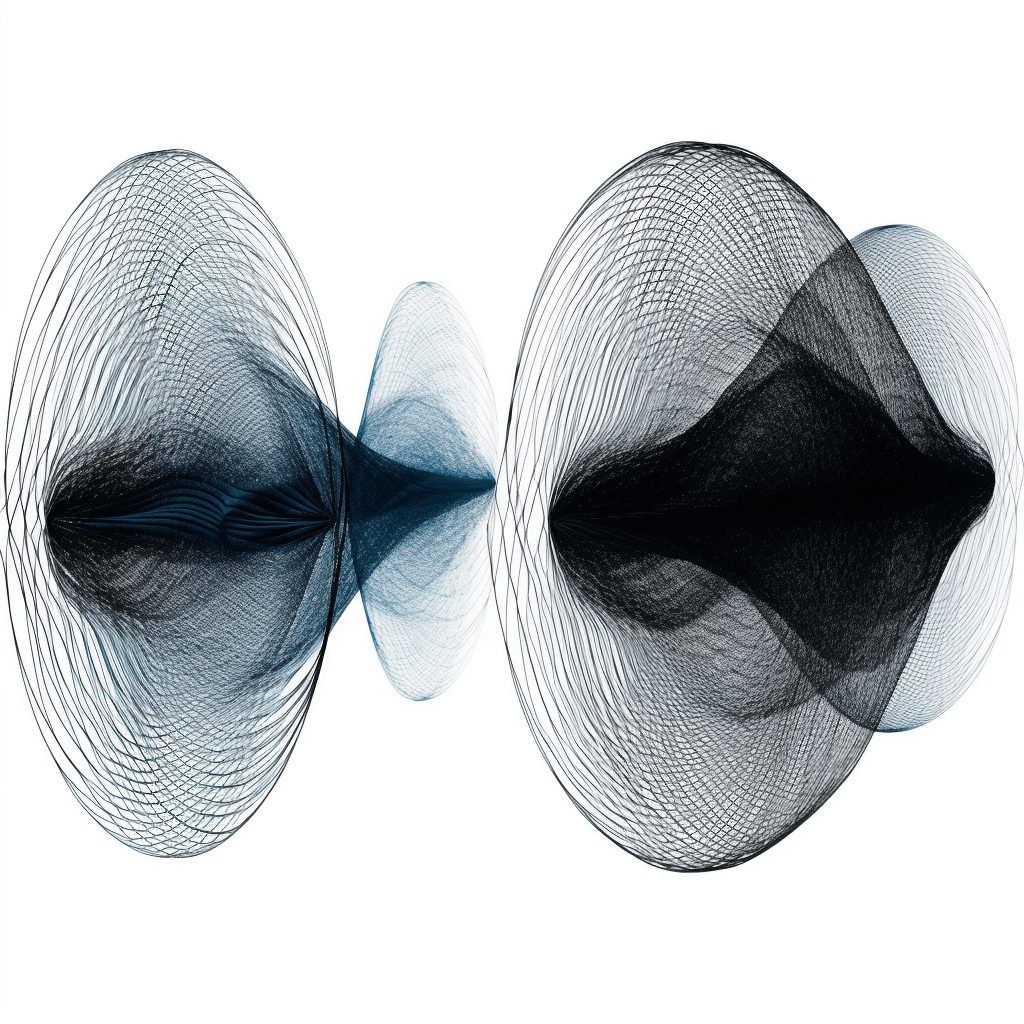
Stay up to date on new AI tool releases
The Road Ahead: Exploring New Techniques and Applications in Audio Analysis
Despite the challenges and risks, the field of audio analysis with deep learning is ripe with possibilities for researchers and practitioners alike. By continuing to explore new techniques and applications, we can unlock the full potential of this exciting field and pave the way for a future in which machines can truly “hear” and understand the sounds of the world around us.
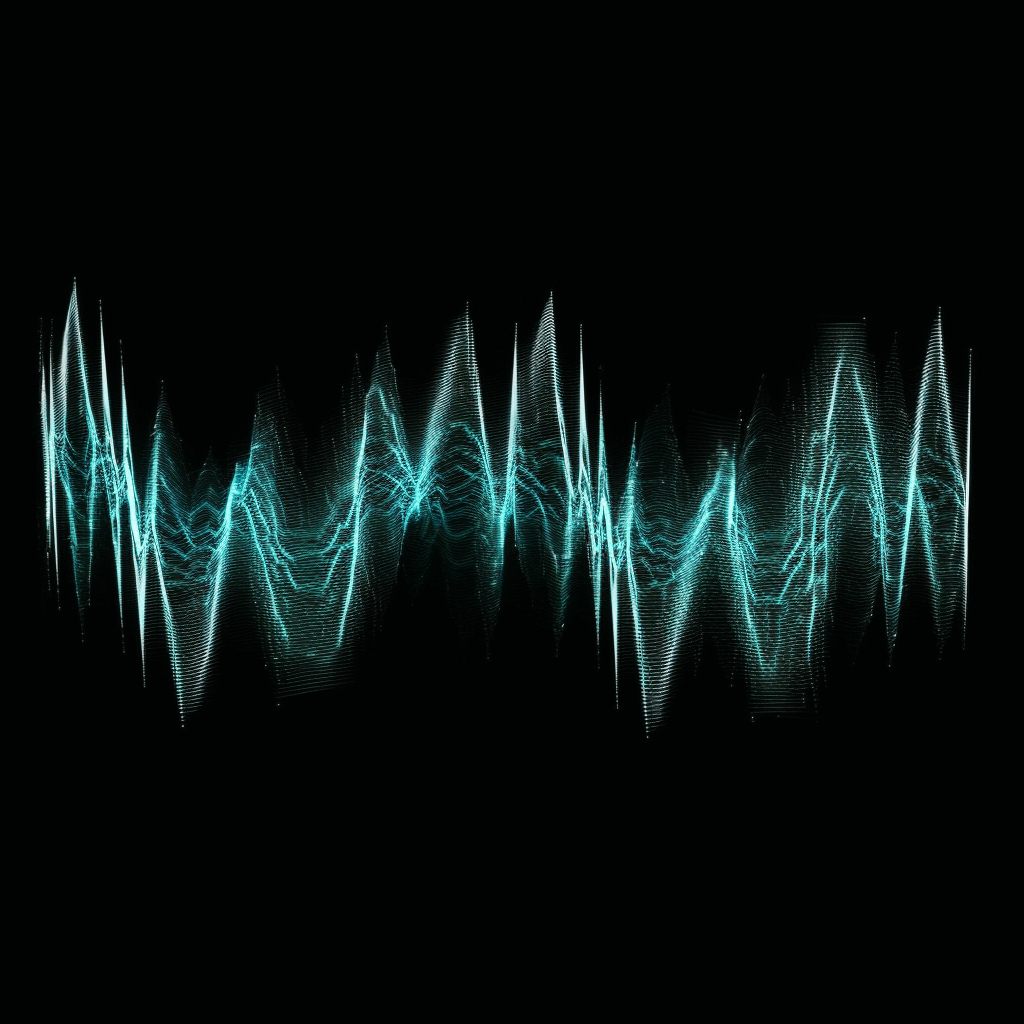
Conclusion
And there you have it, folks – audio analysis with deep learning, from dog barks to door hinges and everything in between. It’s a world of endless possibilities, limited only by our imaginations (and the occasional screeching feedback loop).
But with great power comes great responsibility – we must use our audio analysis abilities for good, not evil. So let’s all agree to not use our AI algorithms to create the next “Friday” or “Baby Shark” (unless we’re trying to start an all-out war with our enemies).
And remember, even though we’re using deep learning techniques to understand the intricacies of sound, there’s still no substitute for good old-fashioned human intuition. So keep your ears open, your mind sharp, and your sense of humor intact – because in the world of audio analysis, you never know what kind of surprises are waiting for you.
Keep reading
How to create Mortal Kombat with AI characters video
The process detailing on how to make a Mortal Kombat…
Read More